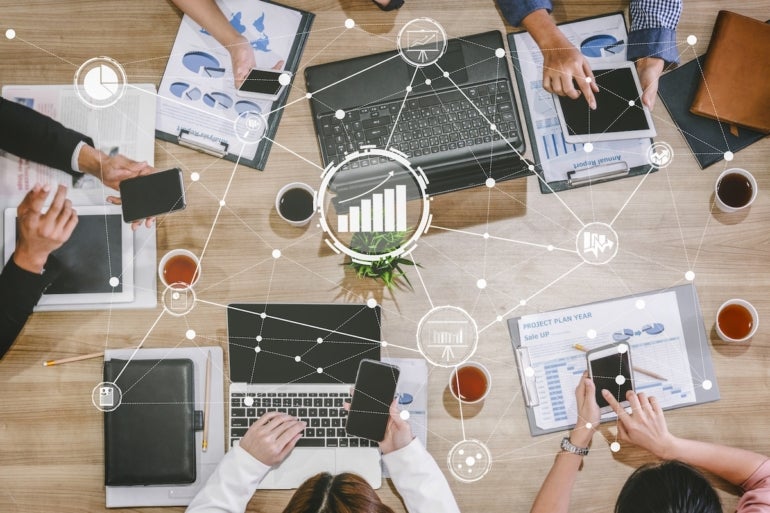
AI adoption is a given in business today — Forrester’s latest Data and Analytics Survey found that three in four enterprises have embarked on the journey of using AI to transform their business.
Now that ChatGPT has barreled into the workplace, AI leaders are seeking ways to take advantage of new generative AI capabilities. AI has become more than just another analytics capability, however; AI leaders are learning that they must also tackle broader questions around ethics, bias, security, regulations and unforeseen outcomes.
To accelerate transformation with AI while addressing trust, tech leaders must rethink their data strategy. This may seem counterintuitive. Wouldn’t you invest more in data science resources and develop data science talent to complement your AI use? Although that is certainly needed, when we talk to any AI leader, they’ll tell you that their first principle is the integrity of the data. Data trains AI; data powers AI in solutions; and data observes AI. You must get your data strategy right to ensure that your AI initiatives bear fruit.
If AI is going to transform your business, your data strategy needs to connect data partners, practices and platforms. Tech leaders must reexamine how data is sourced, how data is used and engineered for AI applications and systems and how governing data ensures that we build trust in AI. Ultimately, to create a system of connected intelligence, companies need a modern approach to data, especially across three competencies.
Jump to:
Strategic data sourcing
Traditionally, data domains are all encompassing and defined by master data concepts such as customer and product. Traditional data domains are monolithic and expected to serve all use cases. While this simplifies data management, it in turn frustrates AI. Data scientists struggle with constrained data domains designed for business intelligence and performance reporting. More information is needed for a machine learning model to execute a task and decision. More metadata is needed to describe an entity, behavior or outcome. And data scientists rely on a variety of data types beyond structured data such as documents, emails, images, video and audio to build AI capabilities.
Today, a data domain is contextual to a business objective, decision and outcome. The scope is specific. AI forces data scientists and data stewards to seek out internal and external data that is relevant, appropriate, of high quality and permissible to use. Modern enterprise data platforms should maintain all enterprise data. Data stewards partner with data scientists to not only identify data from third parties and partners but also to navigate terms, conditions and cost. A marketplace for data enables data science self-service where data, views and policies are ready for consumption and application.
Data operations
Data architecture, engineering and management have historically concentrated on bespoke centralized systems. Enterprise data teams concerned themselves with data warehousing and application databases. Data is moved in batches and copied endlessly. Centralized systems satisfy business requests for a single source of truth, yet they also create complexity, cost and inflexibility.
Going forward, data scientists and data engineers must team up to prepare training data iterative for ML models and, overall, for data science scale. Data engineers are using ML to label, classify and annotate all data to make it ready for data scientists. Data scientists then complete the last hygiene check and preparation of training data. The partnership between data engineers and data scientists has another benefit: Agile practices and CI/CD approaches are shared and coordinated. Each role builds AI components (i.e., models, pipelines and controls). AI is then composable and faster to deploy.
AI governance
Traditional analytics are often deployed and forgotten. Data governance is an afterthought. These practices put AI at a disadvantage. ML is trained by a data scientist but learns and evolves in production applications. Change in AI output and outcomes is constant. And automated AI can quickly scale bias and undesirable outcomes when it goes unchecked and is loosely governed.
AI governance extends data governance in scope and roles. First, introducing a layer of control ensures that all ML, pipelines, data and processing is performing to the service-level agreement. Policies, standards and business logic are federated and contextual to the AI experience rather than the data source and data fabric. The system is transparent with observability and ModelOps capabilities to allow data and ML engineers a window into the data and AI landscape. Second, business stakeholders and decision-makers monitor, test and optimize the data, ML and overall AI to meet AI governance requirements and business outcomes.
AI should no longer be added to digital systems as an afterthought; it defines and drives digital transformation. Data, AI and business professionals all play a role in AI design, development and ownership. A connected intelligence framework can guide tech leaders to ensure that their companies elevate AI to first-class status and, as a result, focus first on their data strategy.
Learn more about key elements that comprise an AI-first data strategy.
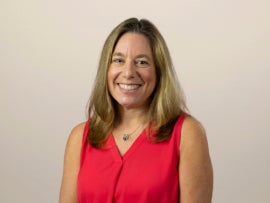
Michele Goetz, Forrester Research vice president/principal analyst, serves enterprise architects, chief data officers and business analysts trying to navigate the complexities of data while running an insight-driven business. Her research covers artificial intelligence technologies and consultancies, semantic technology, data management strategy, data governance and data integration. Michele has over 20 years of experience in data management, business intelligence and analytics.